At HURIDOCS, we develop tools and strategies that make evidence, law and research more accessible to those who promote and protect human rights. We are using the power of machine learning to help our partners at UPR Info with their work of promoting human rights through the Universal Periodic Review (UPR).
Watch this video to learn more:
“It’s really been amazing to see how a technology like machine learning can be applied to human rights and have a real impact.”
–Grace Kwak Danciu, Google.org Fellow and HURIDOCS Board Member
The UPR is the process of reviewing the human rights situation of all 193 member states of the United Nations. Every five years, member states make recommendations to other states resulting in the review of 42 states each year. This annual review can result in up to 8,000 recommendations. UPR Info captures these recommendations in their database, making it accessible to human rights advocates who monitor states’ implementation of their human rights obligations.
UPR Info’s small team has to deal with massive amounts of information while adding, sorting and categorising it by theme. HURIDOCS, through our flagship tool Uwazi, is helping UPR Info with the power of machine learning to better curate this massive body of human rights information.
With the assistance of Google.org Fellows, we used a pre-trained natural language processing model to deal with this challenge. After several tests and comparisons to various other models, we chose BERT, a transformer-based machine learning technique which Google’s search engine started using in 2019. The BERT model is available on the TensorFlow Hub, an open-source software library for machine learning and artificial intelligence. Using TensorFlow, our team worked intensively on refining and adapting the BERT model for the specific context of this domain. The result is a classifier that takes human rights-related text and assigns human rights topics to it. More than 60,000 hand-labeled examples obtained from UPR Info were used as training data.
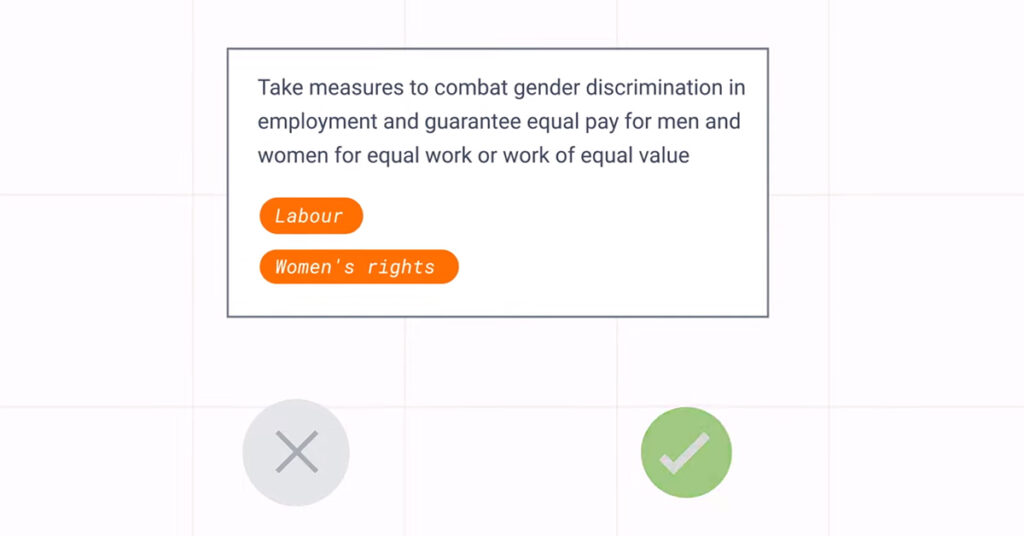
While processing the UPR recommendations, the team from UPR Info has to explicitly accept or reject a suggestion. This process trains the algorithm, ensuring that suggestions become better over time. Before using machine learning, the team from UPR Info spent 2 to 3 months updating their Database of UPR Recommendations and Voluntary Pledges every time new UPR information cycles became available. Now the same task takes them only 1 week. This helped UPR Info clear their backlog so that they can spend less time organising and more time mobilising their tools to help human rights advocates hold states accountable for their human rights obligations.